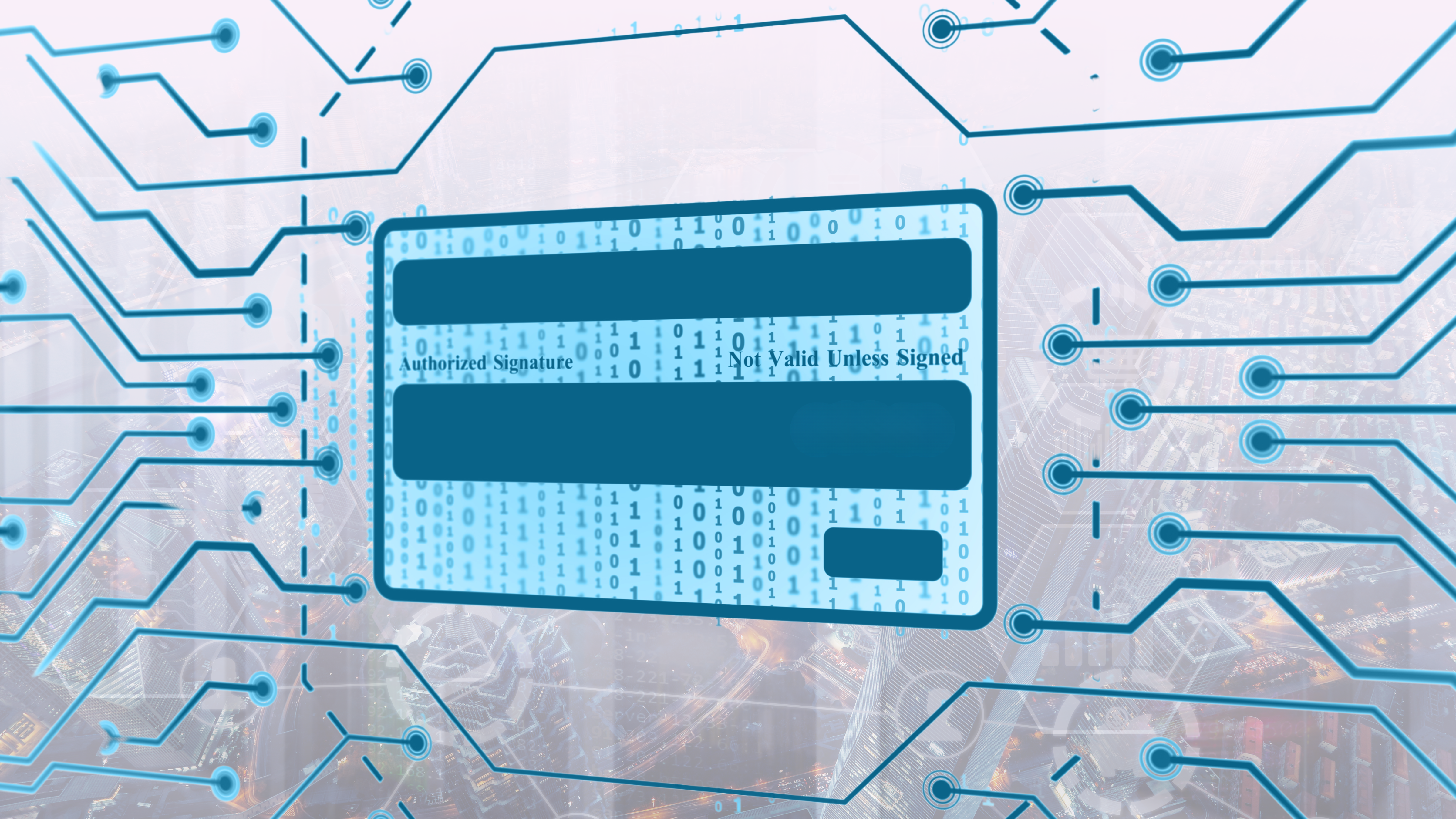
Client case: 83% fewer false positives, lower analyst turnover and higher levels of compliance for international payment processor
Client profile
A European payment processor, monitoring over 1 billion transactions annually and facilitating large financial institutions and retail chains, was looking to enhance its transaction monitoring capabilities in the face of ongoing regulatory pressure. To meet the requirements and ensure compliance, they sought to enhance their existing transaction monitoring engine.
Key results
Quantitative
- 83% fewer false positives
- True positives nearly doubled
- Fewer rules deployed in existing monitoring engine (15 instead of 100+)
Qualitative
- Better model explainability towards auditor and regulator
- Decreased employee / analyst turnover
- More effective investigating
Challenge – too many rules can create blind spots and inefficiencies
While it’s true that rules hold a system together, rule-based transaction monitoring can also create:
- Blind spots. Rule-based transaction monitoring is typically based on formerly known fraud patterns that may no longer be accurate or relevant. After all, financial criminals tend to be agile and dynamic, updating their tactics them to make sure they stay undetected.
- Harmful inefficiencies. Layering rules upon rules increases the complexity of rule-based monitoring over time. This leads to more alerts, most of them false positives. Nevertheless, they must all be checked by your analysts, which can result in a rather rigid system.
The payment processor had 100+ individual Fraud and AML detection rules in place, each with several levels of depth. Such layered complexity and static character made auditability of the rule set low and maintenance time-consuming.
Over time this led to increasing amounts of false positives; a heavy operational burden and regulatory risk. What’s more, analysts were not able to review all alerts, resulting in a backlog. And going through high numbers of false positives all day eventually led to analyst fatigue and high employee turnover.
Eventually, management was urged to undertake action: decrease the number of false positives or hire more analysts to review all alerts.
Solution – automated model generation for Fraud and AML
Sygno Analytics automatically generated a combined Fraud and AML model with a transparent report based on 18 months of the processor’s raw historical data. Its automated machine learning surfaced granular patterns of legitimate behavior (know good, catch bad) in the processor’s raw transaction data and matched these against the processor’s regulatory requirements to generate a holistic detection model.
Process – smooth integration due to open architecture
After setting up our Sygno platform and loading the client’s historical data from their existing monitoring system, we generated new model(s) based on that data. Next, following internal model acceptance, we integrated the newly generated model into the client’s existing monitoring system. Sygno Analytic’s open architecture facilitates this integration smoothly. In the ten following weeks our generated models ran parallel to the client’s existing models to compare performance and finally, we took Sygno’s models into production.
Results – 83% less false positives, true positives nearly doubled
In the end, the client reported 83% fewer false positives, while the effective detection rate (true positives) nearly doubled. On top of the quantitative results, the client also emphasized how they were experiencing better model explainability towards auditors and regulator. Furthermore, rules deployed in their existing monitor engine went down to 15 from 100+. Lastly, by surfacing unknown-unknowns (making the blind spots smaller) and decreasing the clear unnecessary false positives, they could improve the quality of their investigations which resulted in a significantly lower analyst turnover.
Free up your analysts
Eliminate false positives by optimizing your Transaction Monitoring through modeling good client behavior, allowing your analysts to focus on actual critical cases. Would you like to know more about how to achieve that? Feel free to reach out!
Sygno. Know good, catch bad.
We are committed to enhancing efficiency and accuracy in transaction monitoring by reducing false positives and detecting more financial crimes, addressing the critical need for more effective anti-money laundering (AML) and fraud detection in the financial sector. We do that by leveraging advanced machine learning to model good behavior, making suspicious activity stand out.
Our approach generates transparent, explainable AML and fraud models that are accessible to all financial institutions, regardless of your size, are based on your own data, and can be easily integrated into your existing transaction monitoring systems. The automated machine learning solutions we provide are cost-effective, free up your analysts and improve your transaction monitoring by drastically reducing and even eliminating false positives, enhancing your model transparency, and optimizing detection of financial crimes.
Further reading? Try these blogs!
- A Goldilocks Algorithm: detecting anomalies while respecting privacy rules Transaction monitoring that generate excessive false positives risks unnecessary invasion of privacy. Here’s how it can be done differently.
- Case: false positives -83%, better model explainabilityEU payment processor monitoring +1 billion transactions per year and facing regulatory pressure. High false positives, analyst fatigue and employee turnover.
- Navigating AI regulations is more straightforward than you thinkNavigating AI regulations can feel overwhelming and confusing, may even scare you away from adopting AI. But its more straightforward than it seems.