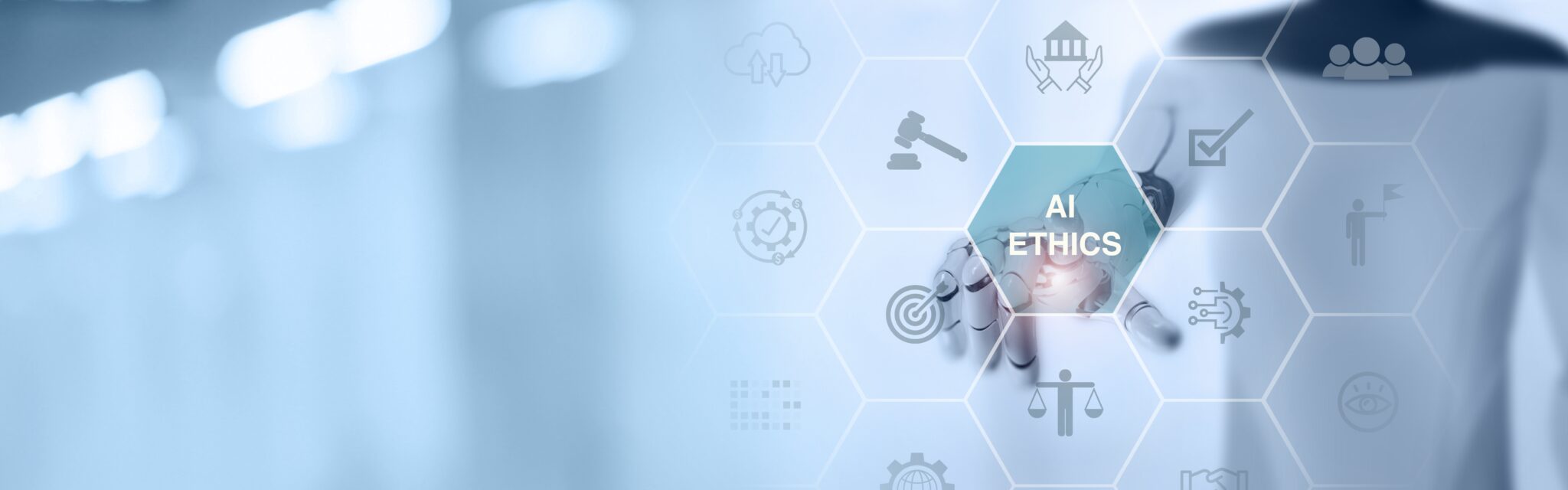
Addressing bias with responsible AI
Understanding how bias can affect model development is important as AI tools are more broadly adopted.
Responsible AI is undoubtedly an important topic. As financial institutions adopt AI and machine learning across their operations, it’s important that these powerful tools are rolled out carefully to prevent biases from affecting model development.
However, some of the concerns around the use of AI reflect an unwarranted fear of the unknown. Bias is a risk in all models. Transaction monitoring is a case in point. Financial institutions that rely on traditional rules-based models often classify entire categories of risk to be too high to manage. This can lead to situations where high-risk sectors or countries are almost fully being excluded.
At Sygno, we believe that our approach has the power to broaden access to finance by enabling FIs to manage risks far more efficiently than is possible using conventional models.
“The main challenge lies in the fact that fraud or money laundering activity only accounts for 0.1% of the data, creating a very unbalanced situation,” says Sjoerd Slot, Sygno’s CEO. “As a consequence of the small number of data points, risk characteristics are typically defined rather rudimentarily and when applied to the larger population result in broad indicators that potentially lead to unethical biases.”
There are two approaches that can help FIs to reduce these biases in their fight against financial crime:
Focus on the 99.9% of non-fraudulent behavior, allowing for far more granular indicators. Use explainable models that can be evaluated for the presence of potential biases. This way AI becomes an enabler to lower bias and unwarranted financial exclusion.