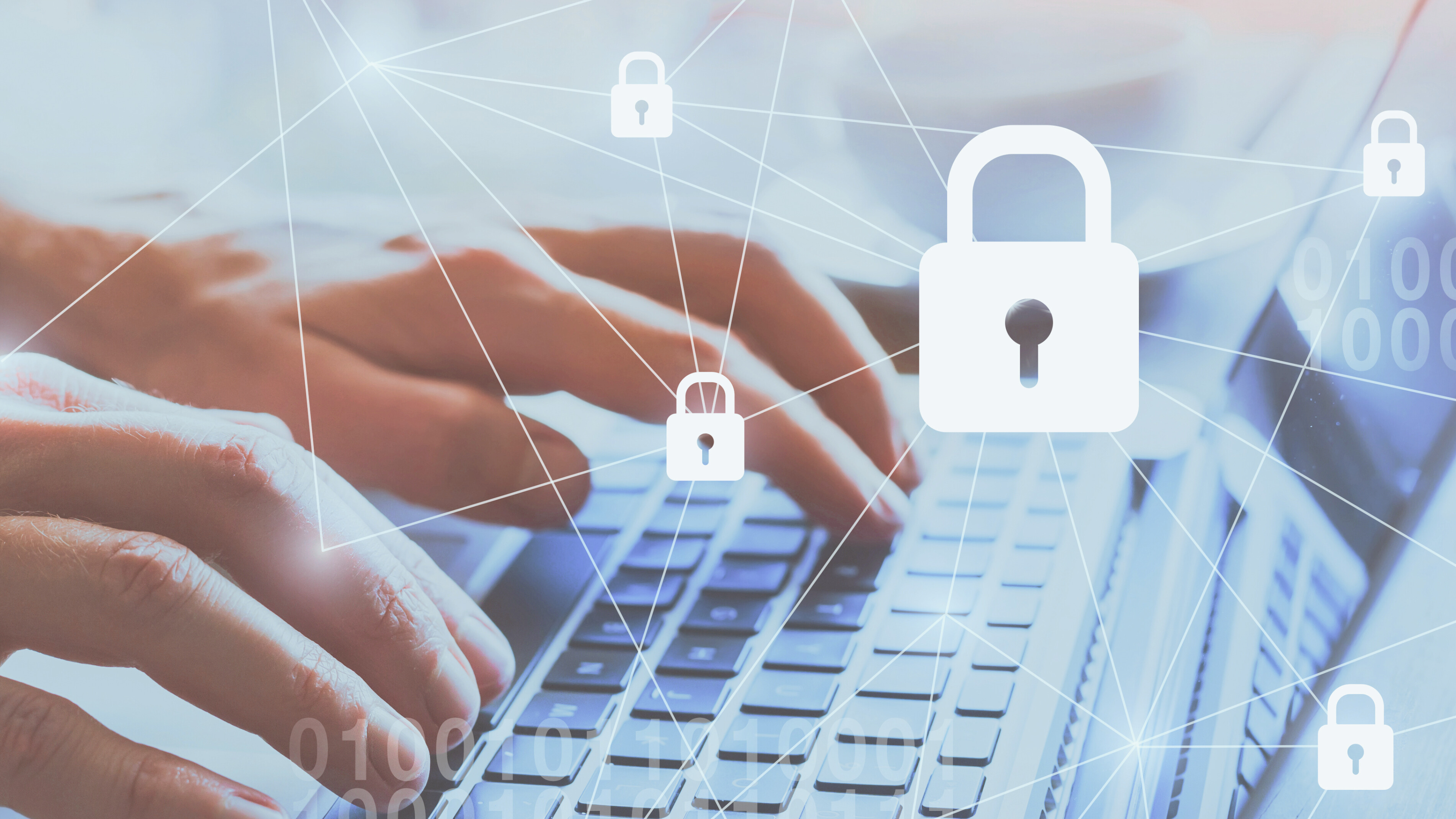
A Goldilocks Algorithm: detecting anomalies in transaction monitoring while respecting privacy rules
Transaction monitoring that generates excessive false positives risks unnecessary invasion of privacy
It’s an ongoing balancing act: protecting customer data under GDPR while meeting Anti-Money Laundering (AML) obligations. With evolving regulations, how can compliance teams effectively monitor transactions and catch financial crime without compromising privacy?
This article explores how responsible AI can create transaction monitoring solutions that hit that “just right” balance.
The privacy-AML balance: a Goldilocks challenge
Excessive false positives in transaction monitoring risk draining resources and unnecessary privacy intrusions. Like Goldilocks, regulators expect financial institutions to get it just right—you cannot just go on fishing expeditions and pry into customer data too much. Yet, you can’t sit back and risk financial crime going unnoticed, either. The challenge is finding the optimal level and balance for transaction monitoring, avoiding both excessive data analysis that violates privacy and insufficient monitoring that misses financial crime.
The Goldilocks Principle, from the children’s story Goldilocks and the Three Bears, is about something being “not too hot, not too cold, just right. It’s a balancing act that encourages management to accelerate opportunities while also ensuring that appropriate guardrails and governance processes are in place—and enforced.”, suggesting a perfect fit and a “just right level of complexity that achieves the best performance on new data.”
The Goldilocks Principle
GDPR vs. AML: navigating regulations in the EU
In the EU, for example, AML rules dictate financial institutions implement systems that “enable the detection of unusual or suspicious transactions”. At the same time, GDPR expects data controllers to demonstrate a “legitimate interest” in processing personal data. Financial institutions are thus required to adhere to AML rules and detect and investigate unusual transactions, while also complying with GDPR’s strict data protection guidelines.
Key considerations include:
- Data minimization and smart, respectful monitoring: Regulators require a balanced approach where data is used only when necessary to meet AML standards without overstepping privacy boundaries. Limiting data processing and structuring your transaction monitoring system to reduce false positives lets your team focus on real threats with confidence.
- Legitimate interest: Ensure there’s a legitimate reason for processing personal data in line with AML objectives. Your institution will need solutions that can detect anomalies without overreaching into customers’ private data, thereby satisfying both sets of requirements.
Effective transaction monitoring happens at the junction of, and in tension with, GDPR and AML provisions, as we have seen in debates around public registers of companies’ beneficial owners, sharing information, and so on. So, how should financial institutions deal with this tension? How can we minimize the impact of privacy while flagging actual unusual and suspicious transactions?
The role of anomaly detection in reducing false positives
Anomaly detection algorithms, when done “just right”, reduce false positives and result in fewer flags to investigate. Modeling and designing your transaction monitoring system for legitimate behavior helps. This allows your institution to detect deviations effectively while minimizing the need for intrusive investigations. As such, modeling and monitoring for legitimate behavior limits the amount of personal data processed, lowers the impact on the privacy of valued customers and leads to you being able to spend more time and focus on actual threats and risks.
Benefits of modeling for legitimate customer behavior:
- Lower privacy impact through reduced data processing
- Save time and resources as analysts focus on actual risks and suspicious behavior
- Enhance detection accuracy for emerging threats with adaptable AI
Moving forward with responsible AI
At Sygno, we help you optimize your transaction monitoring by using responsible AI to generate automated, explainable models that integrate seamlessly with your existing transaction monitoring system. Our solutions empower your team to:
- Reduce false positives by up to 80%.
- Enable transparent, explainable, auditable, and regulatory-compliant models.
- Save operational costs through AI-driven efficiency.
By minimizing false positives, our AI models help your institution remain GDPR-compliant while effectively identifying real financial crime. With seamless integration, you gain immediate accuracy and resource efficiency improvements without costly system overhauls or migrations.
Let’s talk transaction monitoring!
Eliminate false positives by optimizing your Transaction Monitoring through modeling legitimate client behavior, allowing your analysts to focus on actual critical cases. Would you like to know more about how to achieve that? Feel free to reach out!
Sygno. Know good, catch bad.
We are committed to enhancing efficiency and accuracy in transaction monitoring by reducing false positives and detecting more financial crimes, addressing the critical need for more effective anti-money laundering (AML) and fraud detection in the financial sector. We do that by leveraging advanced machine learning to model good behavior, making suspicious activity stand out.
Our approach generates transparent, explainable AML and fraud models that are accessible to all financial institutions, regardless of your size, are based on your own data, and can be easily integrated into your existing transaction monitoring systems. The automated machine learning solutions we provide are cost-effective, free up your analysts and improve your transaction monitoring by drastically reducing and even eliminating false positives, enhancing your model transparency, and optimizing detection of financial crimes.
Further reading? Try these blogs!
- A Goldilocks Algorithm: detecting anomalies while respecting privacy rules Transaction monitoring that generate excessive false positives risks unnecessary invasion of privacy. Here’s how it can be done differently.
- Case: false positives -83%, better model explainabilityEU payment processor monitoring +1 billion transactions per year and facing regulatory pressure. High false positives, analyst fatigue and employee turnover.
- Navigating AI regulations is more straightforward than you thinkNavigating AI regulations can feel overwhelming and confusing, may even scare you away from adopting AI. But its more straightforward than it seems.