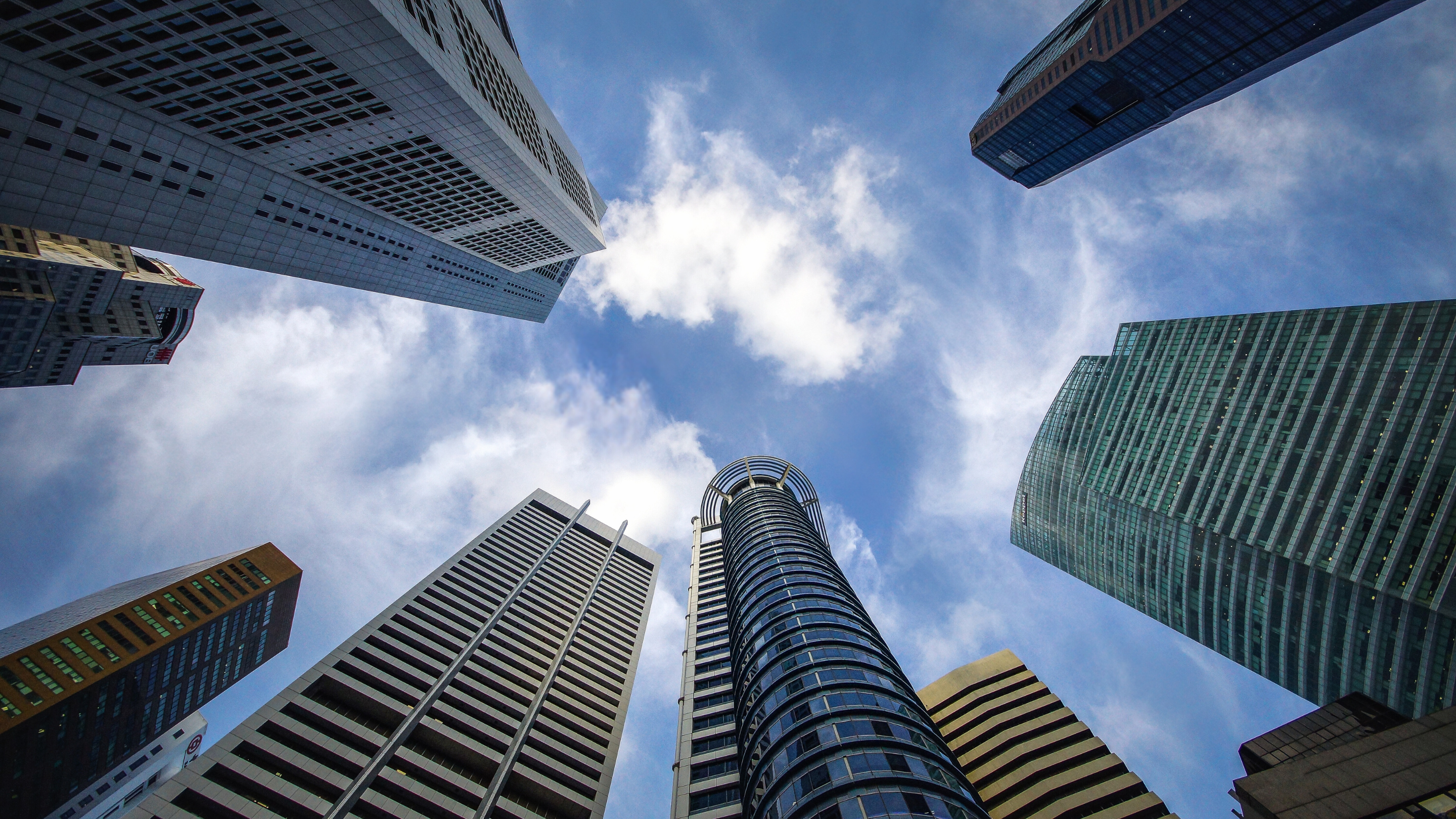
U.S. Treasury’s AI Risk Report: Addressing the growing AI capability gap in banking with Automated Model Generation
While Artificial Intelligence’s rise to mainstream propels our industry forward, we’re presented with some serious challenges. The U.S. Treasury researched some of the major Artificial Intelligence-specific cybersecurity risks in financial services and how to manage them, and published an overview of their findings earlier this year. This article highlights findings that stood out to us and our thoughts on them.
“The rising prominence of AI has increased concern for both potential misuse, manifesting as cyber threats and AI-driven fraud, as well as inadvertent errors, where outputs are mistakenly assumed to be correct.”
Managing Artificial Intelligence-Specific Cybersecurity Risks in the Financial Services Sector – U.S. Treasury AI Report, March 2024 – page 42
The U.S. Treasury presents a series of challenges to address AI specific risk for banking and the financial sector, including:
1) a growing AI capability gap that needs addressing
2) a fraud data divide that needs narrowing
6) explainability of black box AI solutions that needs enhancing
7) gaps in human capital that need bridging.
We believe that these are legitimate challenges. Looking at them from a new perspective could provide practical solutions to help address them effectively and can contribute to building a more equitable, secure, and resilient financial sector. And we’d like to tell you how.
Enjoy the read!
Item 1: Automated Model Generation for addressing the growing capability gap
“There is a widening gap between large and small financial institutions when it comes to in-house AI systems.”
U.S. Treasury AI report, 1st item on addressing immediate AI-related operational risk, cybersecurity and fraud challenges
The U.S. Treasury reports a growing disparity between larger and smaller financial institutions in terms of their AI capabilities. Larger ones generally develop and maintain in-house AI systems more easily and affordably, while smaller ones often don’t have the resources to do the same.
Our thoughts: This seems to assume in-house AI systems are a must-have. A perspective for which a strong case can be made but one that also limits the potential for our sector to move forward as a whole (smaller institutions included). So, let’s explore an alternative perspective.
What if there was a solution for financial institutions of all sizes to access the control and effectiveness of in-house systems, without requiring deep AI-expertise, resources and investments? Enter: Automated Model Generation (AMG) technology. This technology democratizes AI for financial institutions by making advanced capabilities more widely accessible. In doing so it could give smaller institutions a competitive edge without heavy investments in in-house systems, relieve pressure on strained resources (narrowing the growing capability gap) and make our financial landscape more equitable and resilient.
Item 2: Narrowing the fraud data divide: it’s how you use available data that matters
This points to how data is unequally distributed in our sector and holds varying degrees of information, a challenge for smaller institutions trying to deploy AI for fraud detection. Larger institutions benefit from extensive historical data, smaller ones much less so. The finding underscores why this divide needs narrowing to give financial institutions equal access to the benefits AI technology can provide, fairly and effectively.
“As more firms deploy AI, a gap exists in the data available to financial institutions for training models. … Larger institutions hold a significant advantage because they have far more historical data. Smaller institutions generally lack sufficient internal data and expertise to build their own anti-fraud AI models.”
U.S. Treasury AI report, 2nd item on addressing immediate AI-related operational risk, cybersecurity, and fraud challenges
Our thoughts: the large amount of historical data larger institutions have works in their favor, that’s for sure. But the thorough, deep understanding smaller institutions typically have of their customer base can be just as powerful a strength to leverage in detecting anomalies successfully.
It’s again a matter of perspective. Are you looking to detect fraud and money laundering activity by modelling on suspicious (less than 0,01%) behavior driven by the ever-changing tactics of financial wrongdoers? Then, yes. More data is probably better. Whereas if you focus on finding and monitoring expected behavior patterns of legitimate customers (often 99,9%), even limited data proves to be effective because anomalies and potential fraud suddenly begin to stand out, making it easier to spot. E.g. do you focus on the needle or the hay?
In other words, it’s not about how much data you have, it is about how you use the data available to you that matters. Changing your perspective like this, or rather inversing your fraud detection approach, lets you achieve more with seemingly less and proves to be a more accurate and fair detection approach, greatly reducing your false positives.*
*We see improvements in true and false positives for case handling averaging around 80% when deploying Automated Model Generation for our clients, across banks with “larger” data volumes (1B+ transactions per annum) as well as across banks with “smaller” data volumes (200k transactions per annum).
Item 6: How AMG & defragmentation can help to enhance black box AI explainability
It’s still difficult to understand, interpret and explain how, exactly, advanced AI systems make decisions and arrive at conclusions. The process lacks transparency (hence the black box) which the U.S. Treasury flags as cause for concern. Because, we need that transparency for many things; including for training, testing, checking and auditing purposes.
“Explainability of advanced machine learning models, particularly generative AI, continues to be a challenge for many financial institutions.”
U.S. Treasury AI report, 6th item on addressing immediate AI-related operational risk, cybersecurity, and fraud challenges
Our thoughts: whether in-house or outsourced, AI models tend to evolve into deep, complex and opaque structures. This complexity is common with advanced AI models and can make them hard to understand, trust and explain, especially for non-technical stakeholders and regulators.
Automated Model Generation (AMG) and defragmentation techniques can break down some of this complexity, allowing for the development of effective AI models while providing transparency and comprehensibility on how they operate. Simplifying complex models into understandable layers and components like this can shed some much-needed light on black box AI processes.
We find that, when implemented, this approach can help financial institutions comply with regulatory standards, build trust among (non-technical) stakeholders, and facilitate and inform better conversations and decision-making around AI.
Item 7: Bridging the human capital gap
Bridging the human capital gap refers to an increasing shortage of people, talent and technical competence to manage AI risks, particularly in the legal and compliance field. A potential solution presented by the U.S. Treasury included role-specific AI training for employees outside of IT.
“The rapid pace of AI development has exposed a substantial AI workforce talent gap for those skilled in both creating and maintaining AI models and AI users.”
U.S. Treasury AI report, 7th item on addressing immediate AI-related operational risk, cybersecurity, and fraud challenges
Our thoughts: creating and working with AI-models traditionally relies heavily on people. Both in development and maintenance, and regarding ongoing education for AI and Risk/Compliance staff and developing the required expertise in adjacent fields. Add high staff turnover and we find ourselves in a burdensome situation indeed.
But there is a silver lining! Much of the AI work across institutions, whether large or small, is fundamentally similar. It is finding relevant patterns in the data, applying the right model, validating model performance and consistency, and ensuring that models align with risk and compliance objectives and act according to ethical and regulatory standards.
Automating parts of the model generation process and providing understandable tools can reduce dependency on specialized expertise. This, combined with role-specific AI training, could streamline operations, make AI work accessible to more people, and ensure effective AI risk management for banks despite workforce challenges. Simplifying AI development and management like this could be an important link in bridging the growing capability gap regarding technical competence.
Conclusion: embrace automated model generation to address growing capability gap
Wrapping up, the U.S. Treasury’s report underscores some major challenges within the financial sector regarding Artificial Intelligence-specific Cybersecurity Risks. Those include the growing AI capability gap, the fraud data divide, black box AI explainability, and a shortage of technical competence in the workforce.
Automated Model Generation solutions can help tackle these challenges by democratizing AI access, leveraging qualitative data for fraud detection, enhancing model transparency, and relieving some of the strain on specialized staff and technical competence.
Looking forward, banking should continue to embrace such solutions to ensure a more equitable, secure, and resilient future financial landscape. AI developments present our sector with great potential and with the right tools and strategies we can simplify its complexities, manage its risks, make its benefits accessible and navigate its opportunities successfully.
Sygno. Know good, catch bad.
We are committed to enhancing efficiency and accuracy in transaction monitoring by reducing false positives and detecting more financial crimes, addressing the critical need for more effective anti-money laundering (AML) and fraud detection in the financial sector. We do that by leveraging advanced machine learning to model good behavior, making suspicious activity stand out.
Our approach generates transparent, explainable AML and fraud models that are accessible to all financial institutions, regardless of your size, are based on your own data, and can be easily integrated into your existing transaction monitoring systems. The automated machine learning solutions we provide are cost-effective, free up your analysts and improve your transaction monitoring by drastically reducing and even eliminating false positives, enhancing your model transparency, and optimizing detection of financial crimes.
Further reading? Try these blogs!
- A Goldilocks Algorithm: detecting anomalies while respecting privacy rules Transaction monitoring that generate excessive false positives risks unnecessary invasion of privacy. Here’s how it can be done differently.
- Case: false positives -83%, better model explainabilityEU payment processor monitoring +1 billion transactions per year and facing regulatory pressure. High false positives, analyst fatigue and employee turnover.
- Navigating AI regulations is more straightforward than you thinkNavigating AI regulations can feel overwhelming and confusing, may even scare you away from adopting AI. But its more straightforward than it seems.